
This is a weekly newsletter about the business of the technology industry. To receive Tanay’s Newsletter in your inbox, subscribe here for free:
Hi friends,
A few weeks ago, my friend Charles and I brought together a group of tech operators in the AI space for a “Charts and Tarts” event to understand where things are headed in terms of model performance and AI adoption and why we’ve not seen more adoption already. Each person brought a chart related to the topic and we discussed it as a group.
Below are five of the charts from the event which highlight some of the barriers to adoption, how quickly the models are improving, and who is helping fill in the gaps to make adoption faster.
If you’d like to join future events, please email me or DM me on Twitter.
The cost of intelligence is plummeting
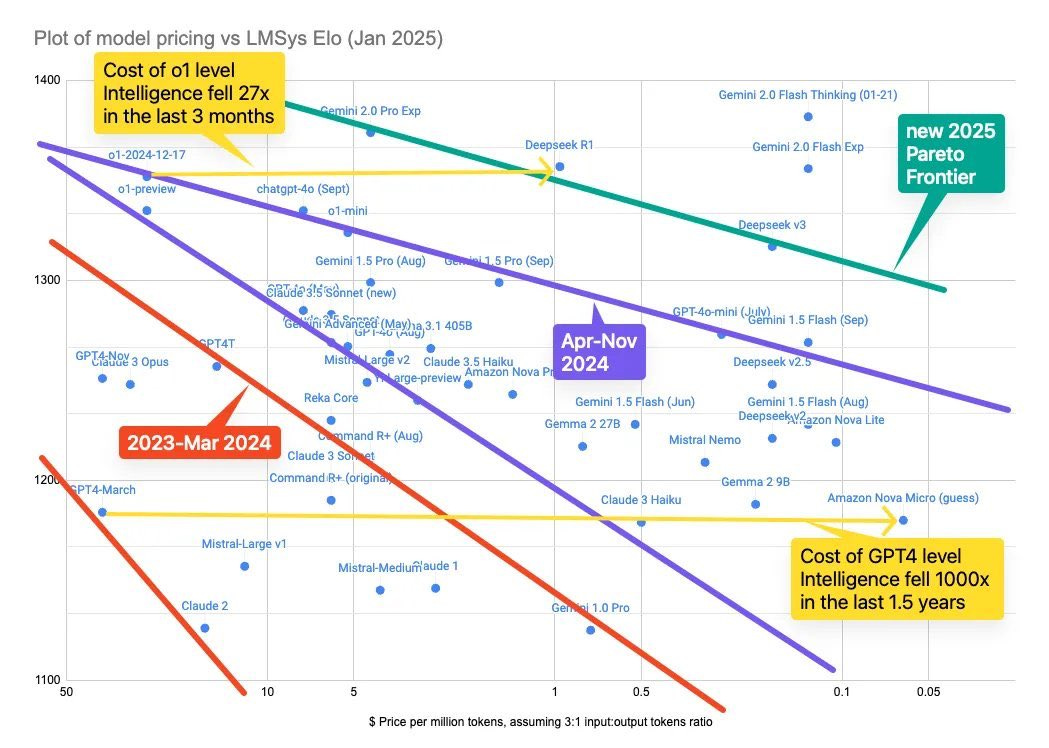
The chart above illustrates the inference cost per million tokens for various models. Just a year or two ago, only cutting-edge models offered any meaningful intelligence—and they did so at a steep price. Companies were hesitant to integrate AI features, worried that the high costs would either hurt their bottom line or force them into aggressive monetization.
Today, however, inference costs have become far less prohibitive. For example, achieving GPT‑4–level performance now costs nearly 1,000 times less than it did just 18 months ago. Similarly, Deepseek’s R‑1 model has slashed the cost of delivering entry‑level intelligence by almost 27 times in just three to four months.
Previously, high costs meant companies could only afford to focus on the most obvious, high‑value applications, limiting experimentation. Now, as the cost of providing intelligence continues to fall—even as model capabilities improve—businesses are free to explore a wider range of use cases and iterate more rapidly on products such as AI agents.
This significant drop in costs will enable companies to integrate AI into the free tiers of their products, potentially unlocking AI for over a billion new users and sparking innovative applications in everyday software.
Looking ahead, two key factors will sustain this trend: the development of increasingly smaller models and the shift toward on‑device inference, driven by advances in chips and infrastructure that allow models to run efficiently on computers, smartphones, and other devices.
Any task whose outcome can be evaluated can be learned through reinforcement learning
This chart is from the Deepseek R1 paper and shows the R1-zero model performance on AIME, a set of challenging math questions for high school students.
It shows model accuracy improving over training steps, with the blue line representing single‐pass and the red line representing consensus‐based sampling strategies. By the end of the chart, the consensus sampling strategy is outperforming OpenAI’s O1 model on the same questions.
Importantly, the R1-zero model relies purely on reinforcement learning without the need for supervised fine-tuning. This shows the power of purely RL based models to continuously improve model performance, though mainly for tasks which don’t require a human to grade and there’s a clear answer a model can reference (like math or coding).
As this technique gets scaled up, we’ll likely see better and better models, especially at tasks with more verifiable solutions. And better models will over time lead to more adoption naturally.
AI products require many building blocks, some of which are still being built out
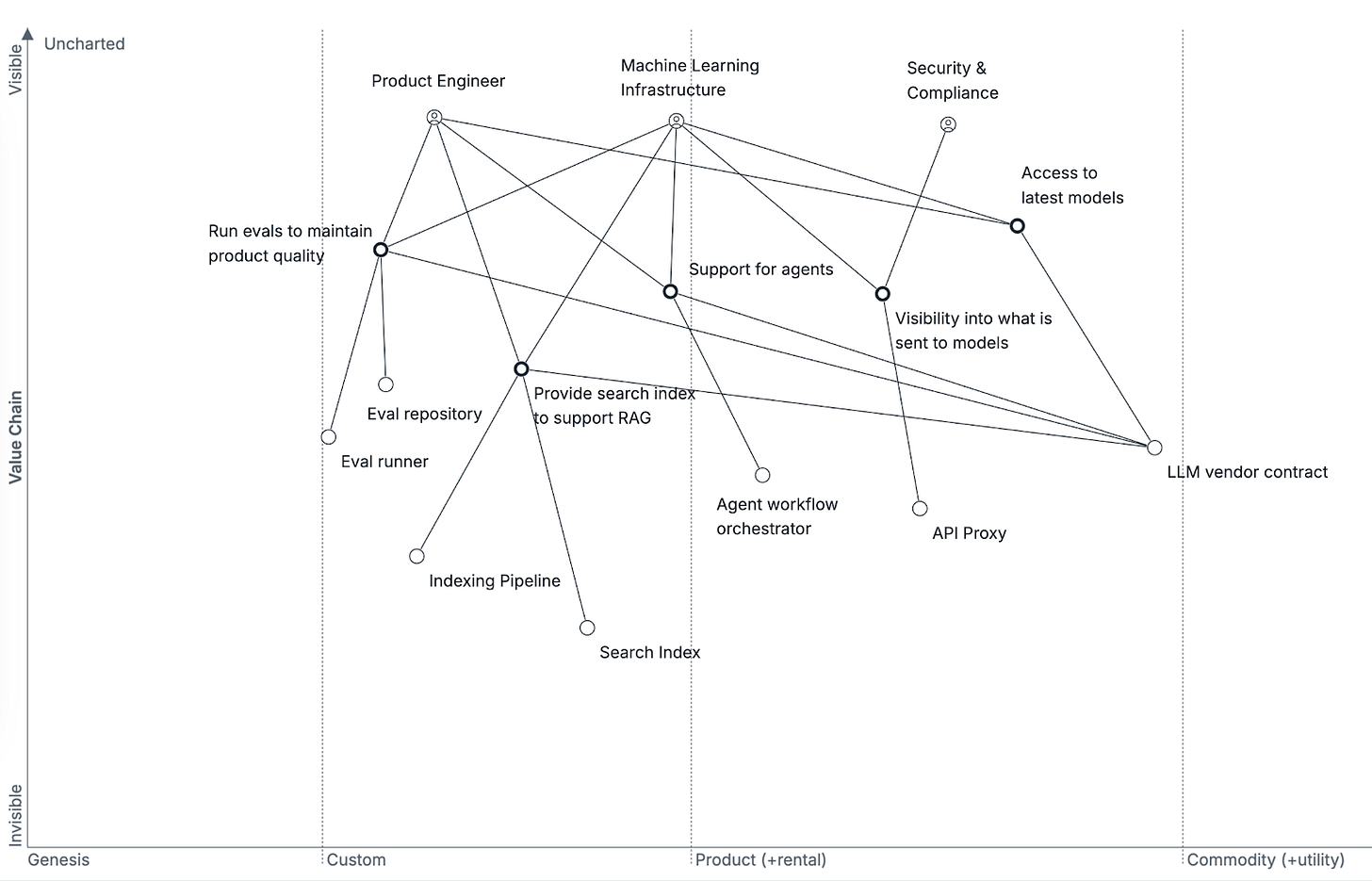
This chart shows a “Wardley map” for LLM products. A Wardley map is a way of visualizing all the pieces needed to create and deliver a product and how far along each piece is in its development. On one side (top to bottom), it shows how visible each piece is to the end user or product. On the other side (left to right), it shows how standardized each piece is.
One of the takeaways from the chart is that building an LLM product today isn’t just about having a good model. They demo incredibly well, but aren’t production ready necessarily by themselves. There are many other parts involved, like creating tools for agents, running evals to test performance (because AI outputs aren’t predictable), and handling security or compliance issues.
And some of these parts are very mature and easy to add, like APIs you can plug into. Others are newer and still being developed. Over time, we should expect most things on this chart to move to the right though as all the tooling becomes more productized/commoditized, which will allow for faster adoption.
If you don’t yet receive Tanay's newsletter in your email inbox, please join the 10,000+ subscribers who do:
Consulting firms are helping enterprise adoption
In addition to the building blocks being built out above, there’s an AI consulting boom happening as enterprises turn to consultants to help them figure out AI.
The chart below shows quarterly “Generative AI” bookings from Accenture, now covering 6% of their total business. If anything though, this may be low compared to other firms. In an article detailing the AI consulting boom last year, the NYT said “about 40 percent of McKinsey’s business [in 2024] will be generative A.I. related”.
These companies are helping businesses decide where to implement AI, how to implement AI and managing some of these aspects today.
Product adoption take time and AI adoption has actually been quite fast
It’s common to hear complaints on why it’s taking so long for people to adopt AI into their work and lives. But that assumes it should be going faster.
In reality, mass adoption of big innovations takes time. It took ~20 years for 60% of Americans to have electricity, and took almost 50 years for telephone adoption to hit the same penetration. That said, it’s been constantly speeding up. The internet took ~10 years to hit 60% of the population.
AI is likely the fastest yet. One reputable survey said 39% of the US population were monthly users as of August 2024, which is much higher than PCs or the internet (both ~20%) after two years.
And so even though some might believe that things have been slow, arguably adoption has been very fast compared to historical technologies.
A bit late to read this- but great post Tanay! :-)